Here’s why you’re struggling with retention — and how AI can help
Stay up-to-date with data-backed insights
Thank you for your submission.
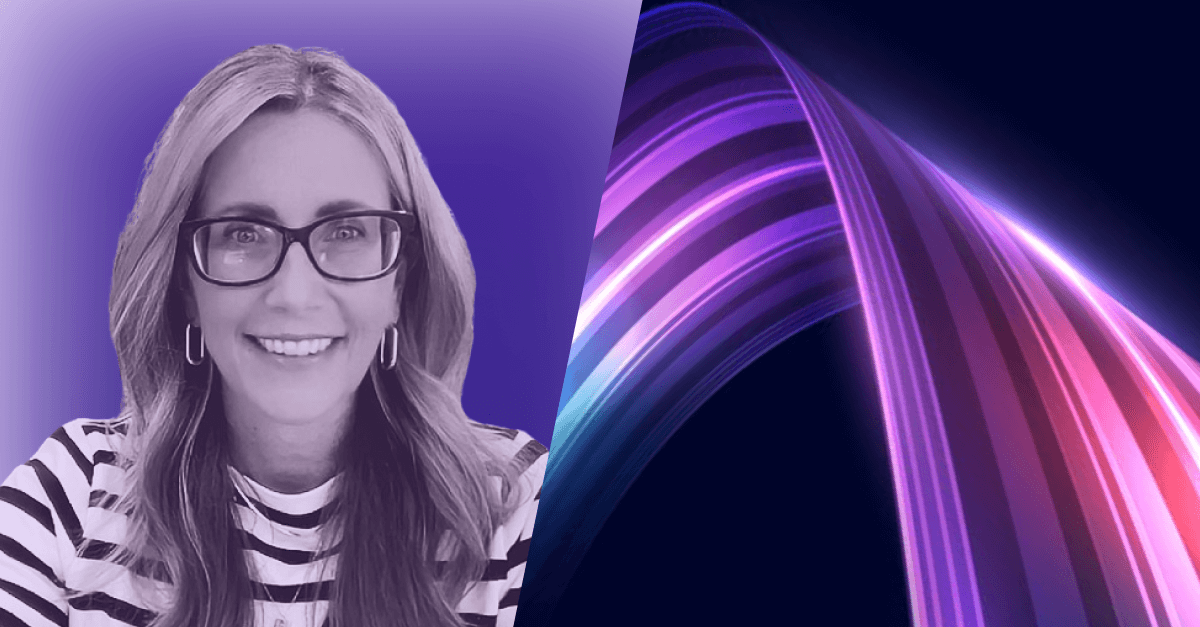
Every SaaS company wants less churn. And in 2024, the way to accomplish that is to move away from a reactive approach to retention.
Improving retention hinges on aligning on customer business outcomes, understanding where there might be friction in the customer journey and providing a clear path to achieving results — but many CS teams rely on inaccurate tools and processes to get these insights. As a result, identifying customer problems becomes an inexact science, making proactive action nearly impossible.
For CS leaders looking to get ahead of retention issues, AI can help. More than just everyone’s favorite buzzword, AI solutions have emerged that capture, synthesize, and extract meaningful insights from a greater volume of more contextual data than ever before.
First, let’s dig into the nature of modern retention challenges.
The three most common pitfalls for CS teams:
- Data shortage. CS leaders need an accurate snapshot of each account path to achieving outcomes and any related retention risk. But this requires unbiased and complete data — which they rarely have.
Leaders often rely on incomplete and fragmented data points, like call notes taken by their team, scant customer feedback, and possibly some product usage metrics, to try and develop an image of the truth. In reality, this is more like panning for gold than accurate risk mapping. Fragmented data leads to an incomplete image of the truth. - Human bias. Moreover, a lot of this data is subject to human bias. CSMs want to believe that their accounts are in good shape (and want to please their bosses). There’s also the insidious recency bias, which leads us to over-value the most recent interactions we had.
- Busyness prevents depth. The busier you are, the more trade-offs you make about how you spend your time — which can mean shallower analysis in certain areas. In a world of incomplete or inaccurate data, you might choose to dive into the wrong area, missing signals about a real customer risk.
How I use AI to address these challenges
- Data completeness. Consider this: Most customer calls consist of ~6,000 words, and the average rep-created call summary is 40–60 words. That means you get about 1% of the data from these calls. How can you realistically communicate progress, identify friction and assess risk based on 1% of the dataset?
AI, on the other hand, uses every single word of that call as the basis for insights. In the blink of an eye, AI can read and interpret all 6,000 words, compare the call content against a global database of customer interactions, and generate insights specific to this customer.
That way, reps can be fully engaged on calls, with no distraction of note-taking or interpretation, and still be able to surface key insights afterwards. - Unbiased. AI doesn’t experience the same biases that humans do. It’s not worried about performance, or unconsciously favoring certain terms while taking notes; it’s giving an objective, unbiased account of customer interactions.
This is particularly true when you use AI solutions that specialize in a single domain (e.g., revenue intelligence). Generalized tools — like those based on GPT — are less likely to possess the domain depth necessary to generate accurate, actionable insights. - Busyness → productivity. Busyness is not the same as productivity. Busyness means working a lot; productivity means working effectively.
AI shows you why customers churn in the first place and how to proactively identify warning signs to get ahead of it. This way, it shows your team where their efforts are most effective — and automates tasks that don’t require a careful human touch.
Churn risk is often the result of not identifying the customers’ critical business outcomes or delivering against those outcomes during the customer journey: this can happen in key customer moments, like when an account transitions from one part of your team to another. By collecting all the relevant information in one common location, AI ensures information consistency during handoffs — preventing the game of telephone that can result in inaccurate, disjointed communication between teams.
AI offers an enhanced customer experience — one where pain points and challenges are anticipated proactively, and customers get as much value from your product(s) as possible.
Fundamentally, CS is a team sport, where sales, CS, professional services, and leadership support the ever-important customer experience. And now, AI is part of that team, too. By acting as a single source of truth, AI creates a connective thread that all teams can use to unify efforts and deliver consistent customer value.
Want more revenue leadership advice and data-driven insights? Subscribe to The Edge.